Rhapsody health solutions experts recently hosted a webinar about the important role semantic interoperability plays in building healthcare artificial intelligence (AI), which was a hot topic at HIMSS23. In the webinar, we discussed observations from HIMSS 2023, why terminology management should be a key part of your interoperability strategy, and tips for building a solid foundation of high-quality data.
Webinar speakers were:
- Panelist Tami Jones, solution consultant, Rhapsody
- Panelist Evgueni Loukipoudis, VP of research and emerging technologies, Rhapsody
- Moderator Shelley Wehmeyer, director of product and partner marketing, Rhapsody
Watch the full webinar or read the transcript, edited for brevity, below.
Skip to:
- What are some key observations and themes from HIMSS?
- The importance of cleansing data for AI and ML models
- Building precision data models
- How do customers use the Rhapsody semantic terminology management platform to build value sets?
- The value of Rhapsody Semantic and Rhapsody Integration
Shelley Wehmeyer: What are some key observations and themes from HIMSS?
Evgueni Loukipoudis: It was not surprising to see a lot of interest in generative AI and everything that has exploded since the end of last year. I was interested in the pragmatic side of things and examples of how AI is being applied in a way that produces results in healthcare.
For example, a health system in Florida applied NLP (natural language processing) on clinical notes, combined with machine learning on observations, medications, and other existing structural data. They discovered that 15% of cases contained unreported conditions, and they were able to realize 2.5 million in revenue. The exciting takeaway is that they were able to use tools they already had in conjunction with AI to immediately realize benefits such as revenue and improved outcomes.
Tami Jones: I saw increased focus on the value of terminology and how it’s applied across healthcare. I had many conversations about the Rhapsody Interoperability Suite, and while the discussions didn’t necessarily start with semantics, they landed there. Semantic interoperability and the terminology management component are essential to the foundation of a healthcare organization. Managing this effectively means having a solid data foundation, then applying AI like ML (machine learning) and NLP.
SW: Rhapsody’s semantic interoperability platform uses generative AI to address the varying terminologies and standards used in medical languages. Evgueni, can you speak to the growing need for semantic interoperability solutions?
EL: At HIMSS, we saw a focus on sematic interpretability of data. With the U.S. common data set, various interpretations, multiple code systems, a lot of structure must be in place before data can be correctly interpreted. An interesting area lies in interoperability between applications.
Many tools, extensions, and systems only work within the right context. That requires definition in good semantic terms, so those who consume and those who provide data can find the right common language. It’s not about normalizing and standardizing, but more about constant transformation and adaptation of conversations that take place within systems and between the humans that use those systems.
The importance of cleansing data for AI and ML models
SW: In last month’s webinar, we discussed how provider organizations move workflows outside of the EHR to drive patient engagement consumer 360 initiatives. Looking at televisits alone, 80% of providers added a digital tool specifically for that purpose in 2022, as compared to 28% in 2019. Across the board, digital health applications are increasing for remote monitoring, clinical decision support, and patient engagement.
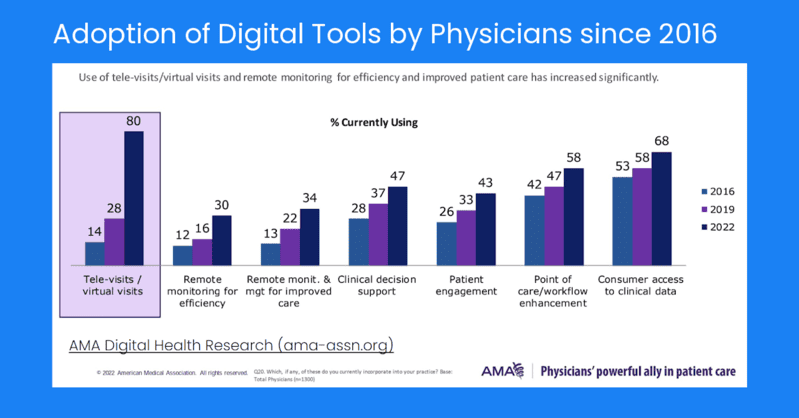
The types, capture, render, and exchange of data in and out of these systems vary. As organizations use data-driven insights to improve operational excellence, an analytical view of what’s going on across systems and specialties means data must be exchanged meaningfully and consistently.
If we define AI as advanced systems designed to analyze data, learn from patterns, and make decisions and solve problems — and definite semantic interoperability as the ability to exchange and interpret information using a shared understanding of the underlying data — it’s easy to see why semantic interoperability is so important. Analytics must build patterns, make decisions, and solve problems from a solid data foundation. As Tami says, we better be singing from the same sheet of music.
TJ: Speaking the same clinical data language is key. Providers and organizations don’t want to guess what a clinical note or concept might mean. But cleansing data before training takes up to 80% of the time. The key lies in how to utilize AI and ML to leverage existing data to enable good decisions about what is meaningful.
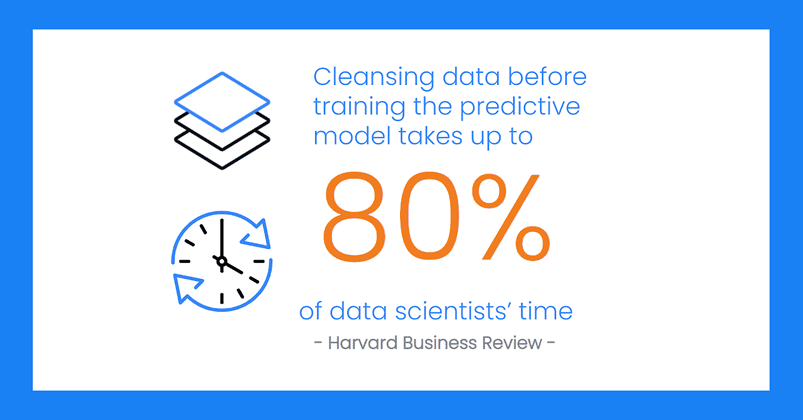
A recent study indicates that 90% of healthcare executives and 98% of providers agree that generative AI is a game changer. Most are planning to pilot (or are already piloting) AI technology this year. So it’s here, it’s now, and it’s coming from both the top and the bottom, because about 40% of all working hours in healthcare can be supported or augmented (not replaced) by language-based AI.
Building precision data models
SW: That’s a key point. We’re working with a customer who’s building precision data models around our semantic product because they are preparing to scale fast. With a team of only three, they knew they needed the right tools to turn over customer data and open data sets as quickly as possible. (Read the case study: From data ingestion to production in less than 30 days: How Zephyr AI uses Rhapsody Semantic to create precise AI models at scale | Rhapsody.)
EL: When it comes to precision medicine, it’s a moving target. You’ll always have to continue improving and learning, especially in the semantic space. The amount of data we collect is growing exponentially, but the information that’s contained in the data is actually growing linearly. This means there’s more data, but less sense is made of it. AI needs to be able to interpret and identify what the provider really needs.
Generative AI is a good tool for creating summaries that will, for instance, justify the usage of a specific data application, test, or exam. But in order for this to function, the semantics problem must be solved. Data cleaning isn’t about removing the noise, it’s about adding additional layers.
Think about the value sets that you can filter through to create a rich vocabulary; you’re not removing the data but clustering it in pieces that help applications and tools get to the right subset. You need to add more layers, because you don’t know what you’re obscuring when you remove data.
TJ: It’s about recycling the data in a different way and putting it in the right buckets of subsets and value sets, so you’re comparing apples to apples. Having the same clinical data language across the board helps build that strong foundation.
You don’t want to remove data; you want to incorporate it. But we have to be able to filter out the noise or incorporate data that says, this is probably not a good idea. You can’t just, for example, remove everything that has a “flat earth” or an “air diet” reference. It’s all incorporated, but there also must be an understanding of its meaning and why it’s there or understand that this is a negation.
How do customers use our semantic terminology management platform to build value sets?
SW: Successful deployment of AI in healthcare and life science organizations must balance the art of possible with the art of practical. Let’s talk tangibly: How do customers use our semantic terminology management platform to build value sets?
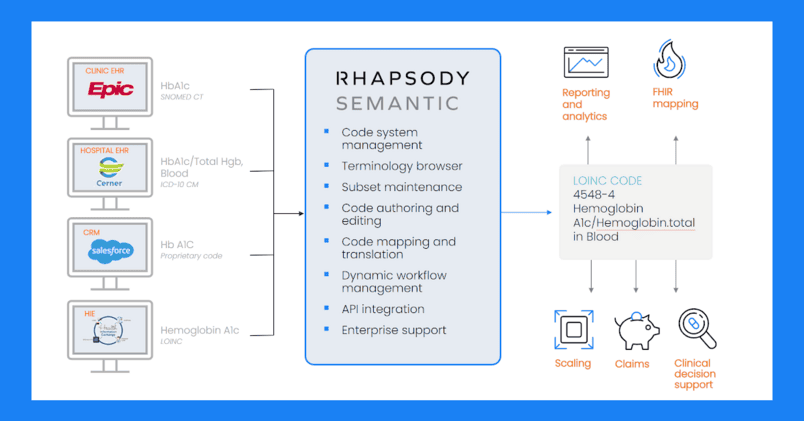
TJ: Our customers use semantic terminology management to filter and find specific things within data. For example, you may want to create sub-hierarchies in a code system. Or you may want to incorporate a specific term into a grouping of concepts that are relevant to a rare disease. Or you may want to use the platform for sensitive data filtering or quality reporting. When you’re creating a meaningful value set for your organization, you’ll put these building blocks together.
Of course, to get there, we must speak and reference the same clinical data language. There’s zero chance that all your systems are sending the same version or the same coding. Rather, they all have a unique, proprietary language, and their own way of speaking it.
Having a tool in the middle, such as the Rhapsody semantic tool, is a key part of the interoperability suite. The real value-add lies in being able to filter and clean the data, put it in the right recycling buckets, settle down the noise, and bring the important things to the surface.
The value of Rhapsody Semantic and Rhapsody Integration
SW: At Rhapsody, it comes back to integration. When our customers deploy Rhapsody semantic terminology management into their environment, it comes with over 100 APIs out of the box to connect to data lakes and downstream systems that tee it up, whether it’s going to a dashboard for reporting or going to a development team to layer on their AI models.
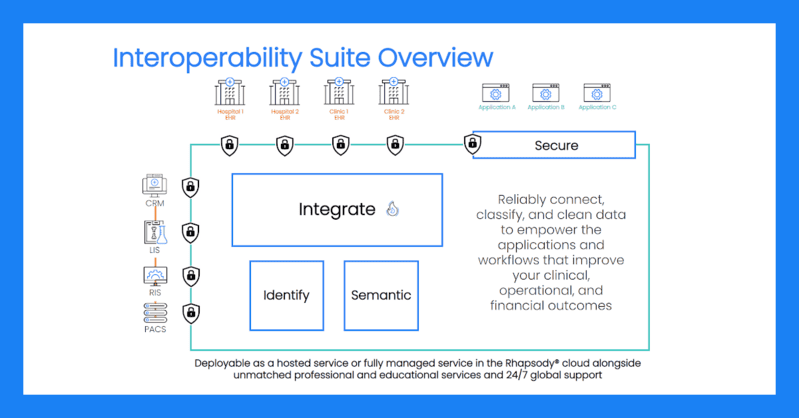
TJ: Rhapsody has our own internal semantic models as well. We embrace the AI and machine learning aspects when we’re applying our own algorithms on top of that data, as we’re going through our mapping processes.
We utilize and leverage this to help our customers to identify potential gaps within their data. We can assess their data to identify what’s non-standard and help them get to a standard code system for that data flow so they can interpret and use that data to its highest extent.
SW: In addition to teeing up a solid data foundation, we’re also applying AI to automate mapping. Our end users — the data scientists and clinical terminologists that use Rhapsody semantic — have the flexibility and adaptability to create their own mappings. We can even create custom terminology sets that can be mapped to or from standard preloaded standard terminologies. This automation allows the system to learn from past mappings and, going forward, to generate with increasing accuracy.
EL: Rhapsody semantic helps organizations to add additional layers, rather than removing them. This ensures they’re allowing a multitude of different uses to accumulate, like different mappings and different code systems.
As for normalization, there is a reason why a certain system uses a certain code and code system, even if it is proprietary. So we don’t always have to force them into a normalized way of working. We can incorporate those layers so that they continue working, while constantly evolving and improving.
In an ideal world, a semantics product will be shared by all customers. We have the tools to make this more collaborative, because the value of AI is not when it’s siloed, but rather within the union of different things.
SW: It’s exciting to think what we can do with this technology to create efficiencies in the future. We look forward to sharing more in upcoming webinars and case studies. Follow our newsletters so you can stay informed.