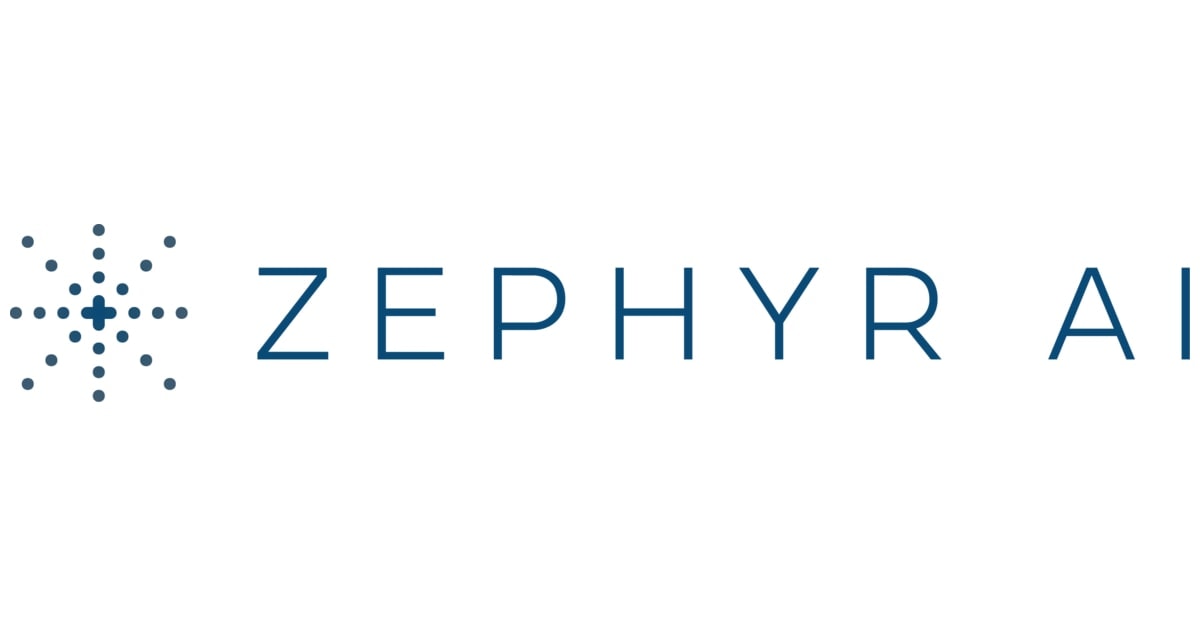
Customer
Zephyr AI
Headquarters
Tysons, Virginia, United States
Website
Organization type
Biotechnology research, artificial intelligence/machine learning, precision medicine
Results
Moving from data ingestion to production in a month, allowing Zephyr to quickly create more precise models that predict optimal therapies for various disease states — leading to better outcomes and lower costs
The customer
Zephyr AI
Zephyr AI combines data science with engineering to help health providers and plans transform prevention, care delivery, and treatment. The Zephyr platform ingests real-world data, then uses transparent artificial intelligence (AI) and machine learning (ML) to develop predictions about outcomes. Zephyr’s proprietary algorithms and predictive analytics make sense of massive data volumes to anticipate risk and prioritize opportunities that improve outcomes and lower costs.
Zephyr combines data from medical and pharmacy claims, EHRs, medical devices, genetic sequencing, cancer registries, molecular clinical data, and more. Some datasets include ICD, SNOMED, CPT, and/or LOINC codes, while other data are semi-structured or unstructured text.
Zephyr works to investigate numerous different use cases and disease states, such as the severity and prevalence of various diabetes complications. Zephyr algorithms examine diagnostic codes, time spent with a provider, and lab results to better predict which patients are most at risk for complications — helping providers target these patients for additional monitoring or treatment. This approach helps improve both outcomes and cost of care.
The challenge
Quickly onboarding vast amounts of new data to start generating meaningful insights
The Zephyr platform ingests data from countless unique data sets, with unstandardized formats and codes. The more high-quality, accurate data that Zephyr can use, the better the outcomes they can provide to their clients. As Amy Sheide, vice president of data platform and partnerships, summed it up, “More data, more better.”
However, Sheide noted, “When the data lands, it’s very disorganized and algorithms can’t make sense of it as is.”
Her colleague, data specialist Samantha Pindak, added, “Clinical data is messy, and nobody uses the same codes. In the clinical data space, EHR records are not standardized. The more data we see in different spaces regarding different diseases, there’s going to be a lot more work with obscure coding systems.”
Before Zephyr can begin applying its machine learning expertise, it must first standardize the data. That means:
- Mapping text strings to standard codes
- Validating any codes that were sent
- Confirming formatting
- Knowledge modeling for implicit features
Zephyr’s “small but mighty” data team of three data specialists needed help. Sheide said, “We’re not going to be passing spreadsheets around. We’re not uploadingand downloading. We wanted our workflow to be in the tool.”
The team also wanted to use its own APIs to protect data security and create efficiencies for their engineering and data science teams.
However, with Zephyr’s plans for rapid growth, the data team must onboard and standardize new customer data to be applied across models — at scale. Since machine learning models are only effective when they receive enough data to learn from, that scaling must happen quickly to enable Zephyr to start generating useful insights for new customers.
“We needed a solution that allowed us to leverage our content management expertise, meaning it needed to be easy and flexible,” Sheide said. This means letting Zephyr’s terminologists and data specialists manage custom code sets, generate their own mappings, and build their own mapping algorithms.
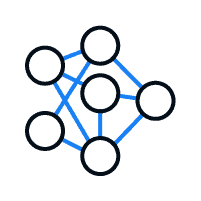
More time spent releasing data-driven insights to predict adverse outcomes and enable proactive care
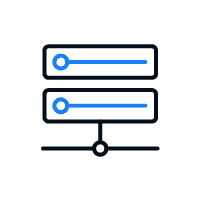
98 billion rows of data processed
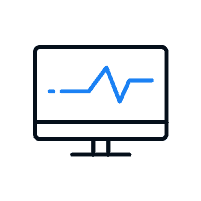
1 month from ingesting data to full production that generates insights
The solution
Rhapsody Semantic to make diverse data useful, faster
Zephyr needed a solution that would allow them to scale to quickly onboard new customers and start generating insights. This required:
- Ensuring data specialists have access to the content they needed
- Allowing them to manage and adjust the content mappings and subsets as needed
- Including with robust workflow management and integration tools that didn’t rely on spreadsheets or custom coding to connect to other systems
The team chose Rhapsody® Semantic terminology management to standardize data at scale. With Rhapsody, Zephyr data can quickly make sense of the data so that it can be passed to engineering to train and test machine learning models.
Now, Zephyr data managers can input custom code systems directly from the source, then translate them to the target without passing around spreadsheets or requiring custom ETL for each data partner . Since the workflows are built right into the tool, the Zephyr team saves significant time and effort with automation.
Rhapsody also allows Zephyr to filter out irrelevant data and curate features of interest, leading to more actionable insights — such as predicting which patients are at the highest risk for an adverse outcome such as a diabetic foot ulcer.
“It goes from looking for a needle in a haystack to simply a pipeline that just matches it for us,” data specialist Nathaniel Tann added. “That’s huge for scalability, being able to easily get the data that we’re interested in from extremely large sources.”
“It goes from looking for a needle in a haystack to simply a pipeline that just matches it for us,” data specialist Nathaniel Tann added. “That’s huge for scalability, being able to easily get the data that we’re interested in from extremely large sources.”
Plus, Rhapsody empowers the Zephyr data team to set user groups with distinct permissions. Each team has exactly the right level of access to do their work, protecting data security.
“The fact that our data managers can go in through the UI and manage the permissions and the code sets and make it operational for engineering is fantastic,” Sheide added.
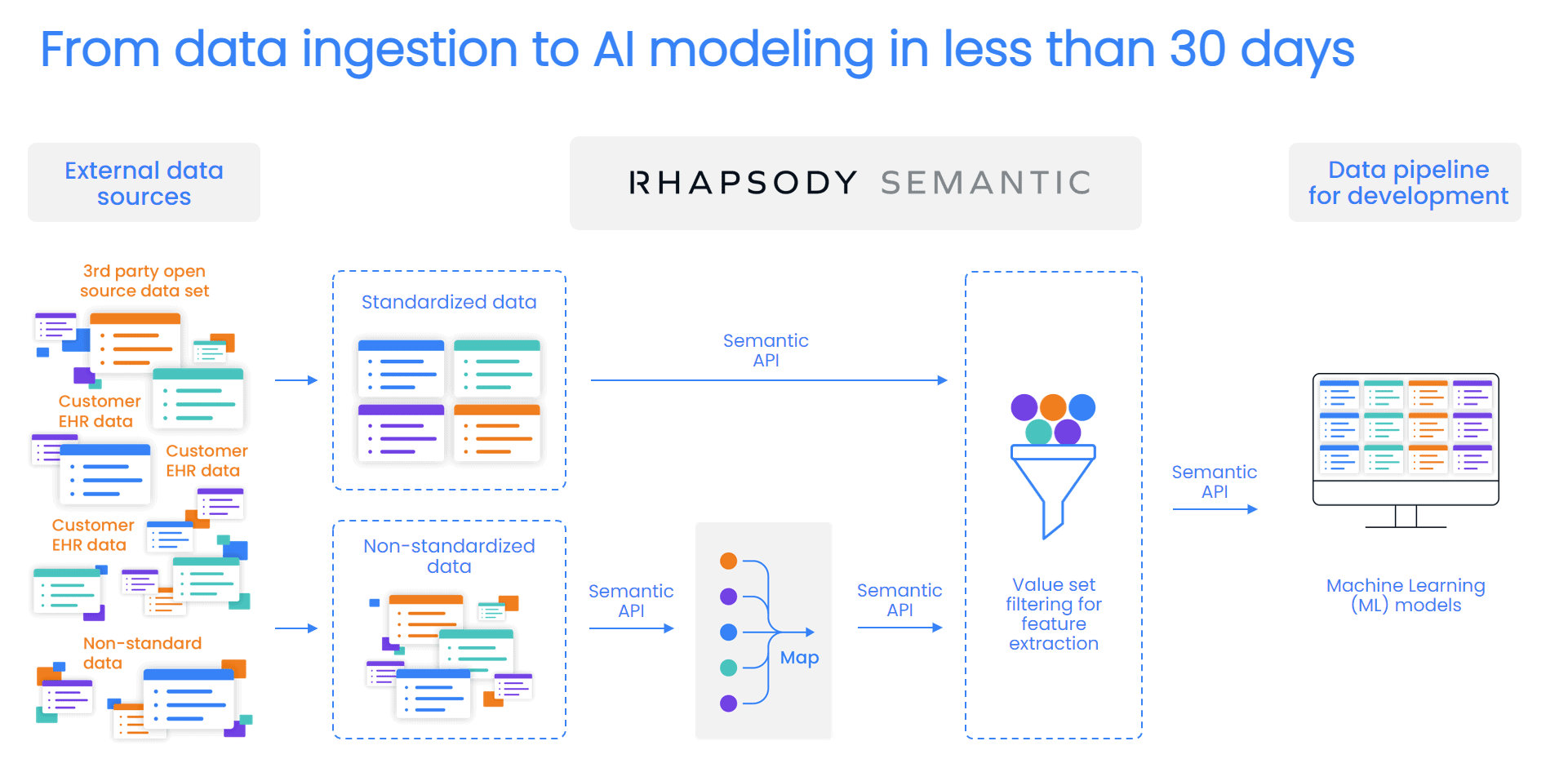
The results
Faster onboarding and insights, streamlined processes
By building a smart data strategy and architecture from the start, Zephyr is now set up to scale. They can deliver healthy data to engineering for development, faster than they could have imagined, resulting in rapidly delivering better insights to their customers. This means that Zephyr can now deliver more value to their customers, faster, while setting a sustainable pace for the future.
“We’re building our data pipelines at a fast pace with a small team,” Sheide said. “We’re getting to production in a month and a half or less, which is very fast for the data sets we work with.”
Being able to clone subsets and apply them to different data sets or sources streamlines the process while “reducing the chatter,” delivering cleaner, more actionable data.
Zephyr has also been able to operationalize its workflows to better manage content between different parts of the pipeline. For example, the additional mappings have provided an additional 35 million lab results from unstandardized text values that would not have previously been usable.
Sheide noted that one member of her data team uploaded a code system, created the properties, and operationalized the API — all in a single day. “That’s really it, how quickly we can wrangle data from different sources to do what we need to do,” she said.
Thanks to Rhapsody, Zephyr is ready to onboard more customers with more data — improving the lives and health of more patients by predicting outcomes faster and more accurately.